Modeling Urban Dynamics for Climate Resilience: Understanding Threats and Vulnerabilities
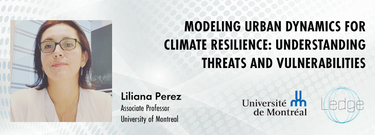
We present here two study cases, the first studies the environmental factors impacting urban beehive health. Honey bees (Apis mellifera) are essential for ecosystems and agriculture, but global colony mortality rates have risen. Our study in Greater Montreal, Canada, with data from 1000 hives, used machine learning to pinpoint factors affecting bee colony health. Higher hive density correlated with lower health, and specific months, particularly September and October, showed a significant reliance on nearby vegetation for hive development. The machine learning model results were used to map predicted honey bee habitat quality, offering insights for conservation and management strategies. The second study located in the capital city of Bogota, Colombia, aims to assess water provision and habitat quality while analyzing their response to land cover changes through the application of the Integrated Valuation of Ecosystem Services and Tradeoffs (InVEST) model. A multitemporal analysis utilized three analogous images to capture land cover changes. Artificial Neural Networks (ANN) were employed to establish relationships between land uses and associated drivers, estimating the probability of occurrence for each land use. Subsequently, the InVEST model was utilized to map the Ecosystem Services (ES) of wetlands, and spatial analyses were conducted to investigate potential correlations between the city's population socioeconomic profile and access to the evaluated ES. This project aimed to position and establish Geosimulation as an important tool for analyzing the crucial implications of policy-making.